Introduction
To bring natural gas from the well to a power plant, a city gas distribution system, or a chemical plant, gas compression is necessary. This involves applications like gas gathering, and flash gas compression near the well, the use of the gas for enhanced oil recovery, or the recompression of gas after processing in a gas plant. It could involve compressing the gas so it can be transported in pipelines, both subsea and over land, or for storage applications as part of a pipeline system. Gas compression is used in processes to liquefy natural gas (LNG). The mainstay for these compression tasks are gas turbine driven centrifugal compressors.
Optimizing the compressor stations can mean a number of different things. The most general definition might be to reduce the cost of transportation or production, either by reducing the expenditures of transporting or processing a certain amount of gas, or by increasing the capacity of a given installation to transport or process gas (Figure 1).
When we talk about cost, we need to recognize that there is a cost for the user or operator of the gas compression station, as well as for the environment and the society. Cost components, besides the cost for establishing the station, may include the cost for fuel, the cost for maintenance, the cost for units not being available for production. The production capacity plays a role, because increasing the production capacity may reduce the per unit of production cost.
This also means that any of these factors is dependent on the efficiency of the compressor or the gas turbine, not as an individual, isolated factor, but rather as an integral part of an overall system.
In this paper, we will evaluate efficiency optimization with examples both at the level of the individual components, but also at the level of an individual compressor station, and at the level of an entire system of interacting compressor stations, as they frequently occur in larger pipeline operations. One of the particular characteristics of most oil and gas applications lies in the fact the operating conditions of the machinery will change on all time scales, be it by the minute, the hour, the day, the season, or over many years.
Equipment level
A typical optimization task for gas turbines would involve the increase of thermal efficiency (i.e. the fuel consumption per unit of power output), and the increase in power density (the amount of power per units of mass flow). Both parameters can be improved by increasing the firing temperature of the engine (Figure 2). However, increasing the firing temperate on an existing engine will have a negative impact on engine life, that is the time between necessary overhauls, and related to that, the reliability of the engine. This effect can be countered by increasing the amount of cooling air for the turbine blades, but that will also reduce the engine efficiency. By being able to very accurately predict local blade temperature, as well as to verify these temperatures experimentally one can use cooling air as miserly as possible. Tools like conjugate heat transfer, irradiated crystal sensor measurement methods, as well as improved materials and coatings can help in this balancing act (Kurz et al., 2017).
Similarly, for the improvement of the centrifugal gas compressors (Figure 3), the change in operating conditions requires not just a higher efficiency (for the compressor, this is the ratio of work that an ideal, isentropic compressor would consume compared to the actual work consumed), but in particular, that a good efficiency is available over a wide flow range. Again, we have two contradictory requirements. CFD tools have allowed us to better understand flow structures in impellers and diffusers, and, together with experimental methods, led to improvements in both operating range and operating efficiency.
Station level
In a compressor station, we may encounter one or multiple gas turbine driven compressors. In many instances, they are different in terms of compressor design point, turbine power rating, and vintage. In many instances, the compressors are sized to meet a certain, well defined operating point, that is to bring a gas of a known inlet condition (gas composition, gas temperature, gas pressure and gas flow) to a defined discharge pressure, for defined ambient conditions (temperature, barometric pressure, and humidity). The reality is that this design point does not exist (Figure 4). Rather, the operating conditions will change. In fact, they are not just variations around the design point. In many instances, the most frequently occurring operating condition is distinctly different from the design point. Large variations in ambient temperature, with their impact on gas turbine power output can move a full load operating condition at high ambient temperatures to a part load operation when it gets colder. Gas turbines tend to lose efficiency when they are operated in part load. Therefore, one of the optimization tasks is to decide, whether the compression task should be accomplished with one or two large units, or a larger number of smaller units.
The example in Figure 5 illustrates the difference: A station with 3 units allow the shutdown of one of the three units during colder temperatures, because the compressor operating range allows for a one third higher flow, while still maintaining good efficiency. The jump in operating conditions for the compressor if the station had two bigger units on the other hand is too big. Thus the station with three units would operate more fuel efficiently. As a side benefit, it would also accumulate less maintenance cost.
To deal with the general uncertainty of the operating conditions, Monte Carlo methods have successfully been used. Since rarely all worst case conditions (highest ambient temperature, highest flow demand, highest engine degradation etc.) occur at the same time, significant size reduction, and fuel consumption reductions are possible (Kurz et al., 2013).
Sizing with uncertainty
For typical oil and gas applications, the operating conditions for the machinery to be purchased is based on predictions and assumptions. As stated above, this leads to actual operating conditions that are different from the original “design” conditions. Yet, the installation still has to meet certain contractual requirements. As an example, a pipeline will have to be able to deliver a certain amount of gas.
Traditionally, the uncertainty in assumptions is addressed by adding margins to the predictions, both regarding the process (for example by assuming the pressure loss for a pipeline with larger roughness, or by sizing a compressor station assuming a lighter gas). Since these margins are additive, in many instances the equipment is oversized, and thus operates at reduced efficiency and costs more than necessary.
A more effective method is to assign probabilities to the input operating parameters such as pipe roughness, gas composition, ambient temperature, equipment degradation, or differences between compressor performance prediction and actual performance. Then, a Monte Carlo simulation (Figure 6) can be performed (Kurz et al., 2013). The use of statistical and probabilistic tools allows to better take the unpredictability of component performance, as well as ambient conditions and demand, into account. Using the methodology allows to design plants that perform best under the most likely scenarios, as opposed to traditional designs that tend to work best under unlikely worst case scenarios. The referenced study (Kurz et al., 2013) was performed for a relatively simple scenario, but the method is not limited, and can easily be adapted to scenarios involving entire pipeline systems, complete plants, or platform operations. New approaches to the discussion of spare units are possible. Based on these considerations, significant cost reductions are possible in many cases (Figure 7, (Kurz et al., 2013)).
Figure 7.
Fuel consumption and equipment size (CAPEX/OPEX), optimized using a Monte Carlo simulation (Kurz et al., 2013).
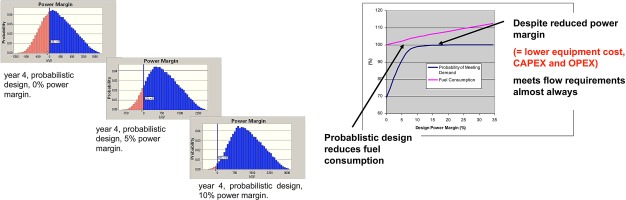
Designing systems
Better understanding and optimization of subsystems (for example, individual compressors, compressor trains, compressor station) allow for optimizations at the overall system level, for example for an entire pipeline, or a network of pipelines.
Optimizing the operation of pipelines (and other compression systems, such as gas gathering systems, or storage systems) requires the accurate simulation of pipeline hydraulics, the turbomachinery systems involved, as well as the behavior of the control systems involved. The time scales involved may require the treatment of transient behavior at the station and the pipeline levels. Notably, these considerations not just evolve around minimizing fuel consumption, but also assuring an acceptable level of availability.
Understanding pipeline hydraulics, and the inherent storage capacity may drive decisions on the number of compressor trains per station, or the requirement for spare units. For example, 3 smaller units in a station may allow to shut a unit down during the colder part of the year, while 2 larger units would not allow this because the compressor operating range would lead to large inefficiencies. This not only optimizes fuel consumption, but also reduces maintenance requirements, and increases the availability (Lubomirsky et al., 2010; Lubomirsky et al., 2016).
The discussion also involves optimizing control strategies. Kurz and Brun (2017) studied the impact of different control strategies (flow control, power control or discharge pressure control) on the fuel consumption, and the operational behavior during transient operating conditions as the occur in pipelines when the line is packed or unpacked in anticipation of a large change in gas demand (Figure 8).
Figure 8.
Load increase in a pipeline system with different control modes for the compressor. Left: Pressure control. Right: Flow control (Kurz and Brun, 2017).
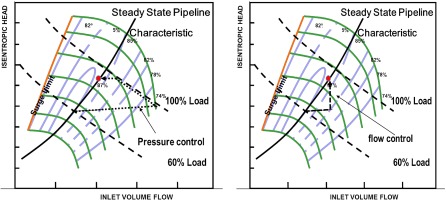
Significant improvements can be demonstrated by combining control functionality, equipment performance, and pipeline behavior. Zamotorin et al. (2018) demonstrated 26% fuel savings as a result of optimizing the operating conditions for the individual compressor station as well as the pipeline in a pipeline system consisting of 5 stations, with a total of 11 gas compressor trains, of various sizes ranging from 6 MW to 15.3 MW.
Ultimately, the concept of a virtual pipeline, where pipeline hydraulics, together with an accurate representation of gas turbine and gas compressor, cooler performance parameters, and an accurate model of the control system and the associated valves, real time performance optimization is conceivable. Systems like that can use data from pressure and flow sensors to improve their predictive capability. Ultimately, combining these systems with health monitoring and predictive maintenance systems can create an environment that can significantly reduce transportation cost.
Macro systems
The future of energy supply and environmental solutions will require the integration of even larger systems: Decisions about reducing emissions should not be made at the level of individual gas turbines. When comparing electric motor driven compressors with gas turbine driven compressors in a pipeline, the electric option would seem to produce lower emissions. However, depending on how the electricity is produced (coal fired, gas fired, renewable etc.), the actual emissions of an electric compressor station, taking into account the emissions generated in electric power generation may be higher than for the gas turbine station, not just regarding CO2, but also regarding NOx, VOC, and particles (Kurz & Brun, 2012). Methane emissions also must be addressed. Key improvements can come from avoiding shutdowns with subsequent station blowdown.
Another area worth mentioning is the discussion on electricity storage, and the integration of intermittently available renewable energy (for example Wind or Solar) into the energy supply systems. As well as looking into new expensive storage facilities based on batteries or compressed air storage, we can take advantage of the natural gas pipeline system and storage facilities. The US alone has over 300,000 miles of inter and intrastate pipelines of various sizes. Hydrocarbons such as natural gas “store” energy and can make the energy available when needed in thermal power plants though the combustion process. There is a huge amount of capacity of stored gas in the pipeline system that is incredibly flexible and, because of the large number of gas producers, any system deficits can be easily compensated with fresh gas from thousands of natural gas wells (Brun and Kurz, 2018).
Future
In general, there are two different paths for improving the current state: One can create more sophisticated, high fidelity models. Some of these are already available, like compressor and gas turbine performance models, or dynamic models of compressor stations, and pipelines. These models can be improved by using precise measurement and sensor data to update these models on a continuous basis, to allow for changes due to degradation, geometry changes, manufacturing and prediction tolerances, or general wear and tear of components.
The other approach would use large amounts of data (“big data”) to detect correlations and to optimize relatively simple models. The problem is that correlations in big data sets do not always describe the real physics of the problem.
Conclusions
By understanding and integrating the operational characteristics of gas turbine driven compressors and the characteristics of the application, compression systems can be optimized to reduce fuel consumption and emissions, reduce maintenance costs, and increase capacity.
This has to involve optimization in the details, such as the cooling flow requirements in the gas turbine, or the aerodynamics of a gas compressor stage, but even more so in optimizing how applications are planned and installations are operated. This holistic approach will offer significant opportunities to reduce the cost of producing and transporting gas, not only for the operators of these systems but also for the society as a whole.