Introduction
According to Gier and Hübner (2005), low pressure turbines (LPT) are responsible for 30% of the total weight and 15% of the total engine cost. Hence, corresponding improvements yield significant benefit for the overall engine performance. Despite of long-term experience, the LPT design is still a challenging task due to increasing requirements on efficiency, weight, durability, manufacturing, maintenance, etc. One of the design trends is to decrease the LPT weight by reducing the number of blades. This results in the design principle of high-lift blades, which are subject to large adverse pressure gradients on the suction side. However, in the low Reynolds number regime, laminar boundary layer separation is likely and may be the primary cause of total pressure loss increase and corresponding efficiency decrease. In the case of non-reattached bubbles, the performance penalties are excessive, a situation wich has to be prevented. In order to maximize the blade loading and to assure a reattachment of the separation bubble, it is crucial that numerical design tools are sufficiently accurate to predict the correct reattachment.The current state of computational fluid dynamics (CFD) for turbomachinery component design is based mainly on three-dimensional RANS methods, as these have a low turnaround time, while often providing satisfactory prediction accuracy. These methods are based on the temporal averaging of the flow equations for the Reynolds decomposed flow quantities in which all scales of turbulence, including transition, are modelled (Wilcox, 1988; Menter, 1994; Langtry and Menter, 2009). RANS turbulence models, in general, can be categorized into several classes. The most known classes are the linear eddy viscosity models (LEVM), explicit algebraic Reynolds stress models (EARSM) and differential Reynolds stress models (DRSM). LEVM are based on the Boussinesq assumption which relates the Reynolds stress tensor directly to the rate of strain tensor of the flow. DRSM, on the other hand, is considered as the most advanced RANS turbulence model. This concept originates from the work of Launder et al. (1975) and completely abandon the Boussinesq-hypothesis. EARSM is obtained through a simplification of the DRSM, and can be perceived as an extension of LEVMs by including the non-linear relation between the turbulence stress tensor, the mean-flow gradient and the turbulence scales.
Despite the great efforts and the successes of recent decades, there is still a lack of prediction accuracy for challenging flow phenomena in turbomachinery (e.g. flow separation, transitional boundary layers, turbulence decay and complex turbulent structures). In particular, LPT’s show significant deficiencies in RANS predictions, since - depending on varying Reynolds numbers (e.g. start or cruise) - different boundary layer states and transition mechanisms can occur (Denton, 2010; Sandberg and Michelassi, 2019). While RANS methods are still the workhorse to perform extensive design variations, with the increase of computational power, high-fidelity simulations such as large eddy simulations (LES) can be performed for few selected operating points and designs. High-fidelity simulations facilitate the understanding of the complex 3D flow physics in the turbomachinery by providing deeper insight into the turbulence field. A profound understanding of the turbulence is necessary to advance the design of improved turbine and compressor blades.
Furthermore, several studies (Comte and Lesieur, 1996; Huai et al., 1997) have confirmed that accurate predictions of transition to turbulence are possible with the LES technique. Due to their reduced modeling share, LES can be mainly used in two ways: either for conducting design-of-experiment-like studies, which provide a thorough understanding of the physical aspects of turbomachinery flow; or for machine learning-based improvements of turbulence and transition models. One aspect of high-fidelity simulations that has not been extensively addressed in the literature is the wealth of information that resides in the anisotropy of the turbulent state. Theoretical methods have existed for a long time, but have not been fully exploited, including the anisotropy-invariant developed by Lumley (1979). Analyzing the anisotropy offers a different view on turbulence, revealing additional information not obviously observed in physical space. In the literature, there are some contributions on the turbulence anisotropy theory, focusing mainly on channel flow, turbulent boundary or wake flow (Antonia et al., 1994; Krogstad and Torbergsen, 2000; Fischer et al., 2001; Schenck and Jovanović, 2002). The flow in turbomachinery has barely been investigated via anisotropy theories based on high-fidelity simulations in the past, with some exceptions focusing on compressor cascades (Yan et al., 2019).
The present investigation aims to improve the understanding of various types of turbulence states by applying the anisotropy invariant theory to a wall-resolved LES (according to Tyacke and Tucker, 2015). For a modern high-lift LPT subject to a low Reynolds number flow regime the anisotropy of the turbulent state with focus on the attached laminar boundary layer, the free shear and the separation bubble are investigated in detail.
Methodology
Describing turbulence anisotropy
Any arbitrary turbulence state can be reconstructed by a linear combination of the three limiting turbulence states, namely: one-component (1C), isotropic two-component (2C), and isotropic (3C). Isotropic 3C turbulence, as the name suggests, forces the anisotropy to be zero, also called “spherical-shape” turbulence. Isotropic 2C turbulence represents a turbulence state in which only two fluctuating components of equal intensity exist (“pancake-shape” turbulence). In contrast, one-component turbulence (1C) is characterized by one fluctuating component which dominates over the other two (e.g., grid turbulence through axisymmetric expansion) and is called “cigar-shape” turbulence. Flows in engineering applications are usually strongly non-equilibrium and anisotropic due to boundaries and high adverse pressure gradients. Turbulence anisotropy can be quantified by the Reynolds stress anisotropy tensor
Testcase
In this study, the highly loaded LPT profile MTU-T161 described by Gier et al. (2010) has been selected. Non-protected experimental as well as RANS (LEVM) and DNS simulation results were first published by Müller-Schindewolffs et al. (2017). The aerodynamic design point and the geometrical parameters of the LPT profile are given in Table 1.
Table 1.
MTU-T161 profile classification.
The three-dimensional model of the MTU-T161, including the diverging side walls, is shown in Figure 1a and the blade-to-blade view in Figure 1b. The profile has been investigated experimentally by Entlesberger et al. (2005) at the University of the Armed Forces in Munich. The measurements include inflow turbulence, profile pressure distribution and wake values. The measured freestream turbulence intensity of the cascade tunnel was increased by using a turbulence grid up to
Numerical method
RANS (LEVM and DRSM) and LES calculations of the above 3D configuration were conducted using DLR’s TRACE solver for turbomachinery flow simulation. TRACE has been used for LES of LPTS application in the past (Leyh and Morsbach, 2020; Morsbach and Bergmann, 2020). The LES calculations in TRACE solve the filtered compressible Navier-Stokes equations using a second-order accurate, density-based finite volume scheme applying MUSCL reconstruction (van Leer, 1979). LES uses a fraction of 10−3 of Roe’s numerical flux (Roe, 1981), which is added to a central flux to avoid odd-even decoupling. The time integration is performed, in this case, using a third-order accurate explicit Runge-Kutta method. The subgrid stresses are computed by the WALE model developed by Nicoud and Ducros (1999).
A one-dimensional characteristic non-reflecting boundary condition implemented by Schlüß et al. (2016) is used at the domain outlet to drive the time- and surface-averaged boundary state towards the prescribed values (static pressure). For the simulation domain inlet, a Riemann boundary condition is applied to set the stagnation pressure, stagnation temperature and flow angles. To reproduce realistic operating conditions, synthetic turbulent fluctuations in the inflow are introduced. The synthetic turbulence generator in TRACE uses the prescribed turbulent length scale and the Reynolds stress tensor components to represent the fluctuation field at the inlet based on superposition of Fourier modes (Shur et al., 2014). These values are adapted from measurements such that the turbulent decay (from simulation inlet to leading edge) matches the experimental decay.
The LES grid consists of 85 × 106 nodes, with a normal wall resolution of
The LES simulation is initialized using the
with an axial chord length
The steady-state LEVM solution in this work was calculated by applying the
In order to extend the comparison, the hybrid SSG/LRR-
Results and discussion
The following discussion is focused on the center plane of the cascade only, although the complete blade height together with the diverging endwalls was covered by the simulation. In this way, the 3D effects from the endwall regions are also accurately captured. These effects have a significant impact at midspan flow, especially due to the large endwall divergence.
Comparison to experiment
Prior to a detailed investigation of the turbulence and anisotropy behaviour, the blade loading and the wake loss are evaluated and compared to the experimental results.
Figure 2a shows the isentropic Mach number (Mais) distribution, which is determined by the static pressure, around the profile. The LEVM results match very well with measurements at the leading edge, trailing edge and the aft pressure side. On the suction side, the Mais-distribution starts to deviate at
Figure 2.
Comparison of averaged quantities of LES, LEVM, DRSM and experiment. (a) Mais distribution at 50% span. (b) Total pressure loss coefficient at x / l ax = 1.4 .
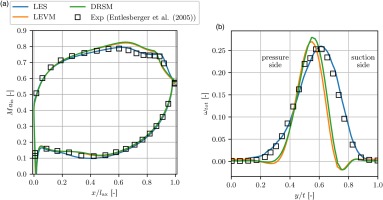
The deficiencies of both RANS models to accurately predict the pressure distribution and the flow loss may be tracked back to the non-adequate reproduction of the turbulence effects in the separation and wake region. Since the separation point is in the laminar state, the turbulence is generated at the outer part of the separation bubble, where strongly anisotropic characteristics are present, as will be shown later. These characteristics are also propagated into the wake. The results of Figure 2 show that even DRSMs (SSG/LRR-
Separation and transition to turbulence
It has been shown Coull and Hodson (2011) that two mechanisms dominate in LPT applications under elevated free stream turbulence (FST) conditions (
To facilitate further discussion, a body-fitted coordinate system is introduced for the suction side region of the profile, with coordinate s starting from leading edge running to trailing edge and coordinate t representing the wall normal direction (Figure 3a). As such, we performed a transformation to the new coordinates for the region of interest on the suction side. In this area, velocities and Reynolds stresses are transformed to the new coordinate system where the indices 1, 2 and 3 indicate the wall parallel, wall normal and spanwise direction. tmax characterizes the edge of the wall normal coordinate t.
Figure 3.
Turbulence in the blade passage obtained by LES. (a) Turbulent kinetic energy (logarithmic scale). (b) Componentality of Reynolds stress anisotropy tensor.
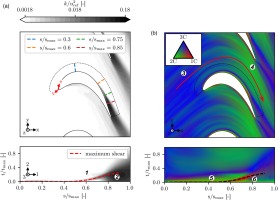
The suction side boundary layer around the MTU-T161 is characterized by the separation-induced transition mode. As shown in Figure 3a, the suction side boundary layer separates at around
Turbulence anisotropy
To get a better overall understanding of the turbulence state, Figure 3b shows the componentality contours in blade-to-blade view. To do this, the methodology of Emory and Iaccarino (2014) is used, by mapping the weights
The near wall area of the profile is dominated by 1C turbulent state, in this case streamwise Reynolds stress. The 1C layer (red color) spans from near leading edge to the separation point. After the flow starts to separate, the prominent 1C turbulence state is stretched along the line, indicating the maximum shear above the reverse flow region.
The second region of interest is the 2C area directly above the 1C layer next to the wall (region 5
In the separated region
In summary, Figure 3b demonstrates the complexity of the investigated flow and indicates that nearly all possible states of turbulence occur in different regions of the suction side. At the same time, this explains to some extent, why most of the RANS eddy viscosity turbulence models (e.g. standard
After looking at the composition of the Reynolds stress anisotropy tensor of the flow, subsequent figures illustrate relevant turbulence states in respect of the contribution of the different fluctuating components.
Figure 4 shows contour plots of the normal and shear Reynolds stresses, normalized by the mean outlet velocity (uref). Superimposed are the inflection line
Figure 4.
Skin friction coefficient c f and Reynolds stress components (with logarithmic scale) along the suction side obtained by LES. (a) Skin friction coefficient c f . (b) streamwise Reynolds stress component u 1 ′ u 1 ′ ¯ / u ref 2 . (c) wall normal Reynolds stress component u 2 ′ u 2 ′ ¯ / u ref 2 . (d) spanwise Reynolds stress component u 3 ′ u 3 ′ ¯ / u ref 2 . (e) shear Reynolds stress component − u 1 ′ u 2 ′ ¯ / u ref 2 .
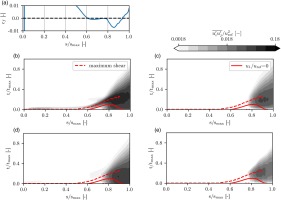
One should note that identifying a reattachment point or line, based on the skin friction plot, is justified for a steady laminar separation bubble. Whereas in transitional and turbulent separation bubbles, the instantaneous flow field is highly unsteady and varies continuously over time. The definition of a reattachment point could therefore be misleading. Here, the definition is based on the time-averaged velocity distribution. Looking at the streamwise Reynolds stress component
Both wall normal
Wall normal profiles of turbulence
After investigating the streamwise evolution of the anisotopy and Reynolds stresses, the following figures present with the Reynolds stress distribution perpendicular to the wall. As in Figure 3b, to get a better overall understanding of the turbulence state, the componentality contours are visualized by mapping the weights
Starting from the front part of the suction side in Figure 5a, in which the flow accelerates and a laminar boundary layer exists, a mixture of
Figure 5.
Reynolds stresses (lines) and the corresponding componentality of Reynolds stress anisotropy tensor (colorbars) along wall normal lines at four defined streamwise positions as in Figure 3a obtained by LES. (a) s/smax = 0.3. (b) s/smax = 0.6. (c) s/smax = 0.75. (d) s/smax = 0.85.
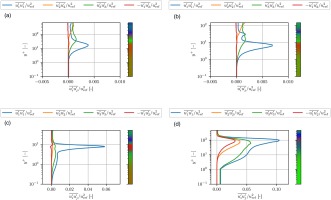
Moving further downstream (Figure 5d), all Reynolds stress components increase and reach their maximum level at
Moving towards the edge of the boundary layer, the
Comparison to RANS
Figure 6 demonstrates the difference in the prediction of Reynolds stress components between RANS (both LEVM and DRSM) and LES at
Figure 6.
Comparison of Reynolds stresses (lines) and the corresponding componentality of Reynolds stress anisotropy tensor (colorbars) between (a) LEVM, (b) DRSM and (c) LES at s/smax = 0.85.
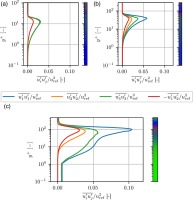
The numerical results show that the underestimation of Reynolds stress is a significant factor in the unsatisfactory prediction of separation. These results emphasize the need to improve the prediction of reverse-flow speed in separation bubbles by LEVMs and DRSMs.
Conclusion
An investigation of the low-pressure turbine cascade MTU-T161 via LES with respect to the turbulence anisotropy is presented. The flow at low Reynolds number 90 × 103 shows a laminar separation bubble which undergoes transition into turbulent state and reattaches close to the trailing edge. The comparison between RANS (LEVM and DRSM) and LES with respect to isentropic Mach number distribution on the profile and total pressure loss in the wake show clear deficiencies of the RANS prediction and a very good agreement of the LES prediction to the experiments. The turbulence state in various regions on the suction side is analyzed with focus on the components of the Reynolds stress anisotropy tensor. Using the barycentric map, it can be concluded that the flow over the LPT suction side includes nearly all possible states of turbulence occurring in different regions. It can be seen that the flow near the wall is governed by one-component anisotropy state, followed by a two-component layer above. The near wall area develops the two-component turbulence within the separated region. In the final stage, the fully developed turbulent state is identified by decreasing the anisotropy in the velocity fluctuations. The separated shear layer above the separation bubble changes from two-component to one-component and subsequently to three-component state. The passage flow also undergoes a shift from three-component isotropic behavior, entering the passage, to two-component turbulence during the large acceleration and flow turning inside the turbine passage.
Finally, LEVM predictions show a nearly isotropic turulence prediction in the separation region, clearly demonstrating the inability of this approach to reproduce the strongly anisotropic turbulence characteristics. This is considered to be the main reason for poor RANS predictions of Mach number distribution and total pressure loss. DRSM results show slightly anisotrop behavior in the boundary layer and separation bubble. Nevertheless the magnitude of Reynolds stress components are still underestimated comapred to LES. The mean flow quantities in the middle section are not captured better than LEVM via DRSM. The present analysis underlines the complexity of the flow in relevant turbomachinery applications, even in two-dimensional mid-span flows. In particular, the turbulence anisotropy seems to be the key element for accurate flow predictions and reliable blade design. This aspect needs more attention in future research and development work, both in turbulence modelling and aerodynamic blade design. Such analysis provide insights for turbulence modelling community to come up with new anisotropic models or turbulence model extensions. These results show that there are still deficiencies in modeling the Reynolds stress transport equation, which need to be further analyzed and corrected so as to improve the current models. This can be done based on novel machine learning techniques.
Nomenclature
Acronyms
RANS
Reynolds-averaged Navier-Stokes
LEVM
Linear Eddy Viscosity Models
DRSM
Differential Reynolds Stress Models
EARSM
Explicit Algebraic Reynolds Stress Models
LES
Large Eddy Simulation
STG
Synthetic Turbulence Generator
TRACE
Turbomachinery Research Aerodynamic Computational Environment
KH
Kelvin-Helmholtz
WRLES
Wall Resolved Large Eddy Simulation
LPT
Low Pressure Turbine
LE
Leading Edge
TE
Trailing Edge
CFD
Computational Fluid Dynamics
TKE
Turbulent Kinetic Energy